Plenoptic PNG: Real-time Neural Radiance Fields in 150KB
Jae Yong Lee1, Yuqun Wu1, Chuhang Zou2, Derek Hoiem1, Shenlong Wang1,
Abstract
The goal of Plenoptic PNG (PPNG) is to encode a 3D scene into an
Training
Training PPNG models can be done with a CUDA capable devices. We implemented PPNG 1, 2 and 3 on tiny-cuda-nn, and ported on Instant-NGP platform. Typically, the training takes around up to 5 minute (PPNG-3) to 13 minutes (PPNG-1) to complete. We provide code to translate trained model weights (.ingp files) into customized format (.ppng files). The translation is does not require huge computation and is done near instantly.
Usage
Once trained and translated, PPNG can be easily integrated to browsers supporting WebGL 2.
All Demo pages are implemented based on the custom component ppng-viewer
that can be used as follows:
<ppng-viewer src="/path_to_ppng_file.ppng" width="400" height="400" ></ppng-viewer>
Real-Time Demo
We provide real-time demo of PPNG on various datasets. For each page, we have 3 different models with different quality and performance trade-offs.
- P1 represents PPNG-1, our lightest, CP-decmposed version of PPNG-3 (with 128KB model size).
- P2 represents PPNG-2, an intermediate, tri-plane decomposed version of PPNG-3 (with 2.46 MB model size).
- P3 represents original PPNG-3 without any decomposition (with 32.8 MB model size).
Each model has RLE encoded occupancy grid cache which are around 15~100 KB for object datasets and 400~500 KB for 360 datasets.
(Note that P3 is not available for some datasets scenes due to size limits in github pages.)
To demonstrate our efficiency, we provide 2 different ways to load the model.
All Objects section loads all objects in the dataset at the same time. It will load 4 to 8 objects simultaneously depending on the dataset.
(Warning! This may take a while to load for P3!)
Single Object section loads single object in each dataset with larger render size.
All Objects
Loads all objects in the dataset at the same time!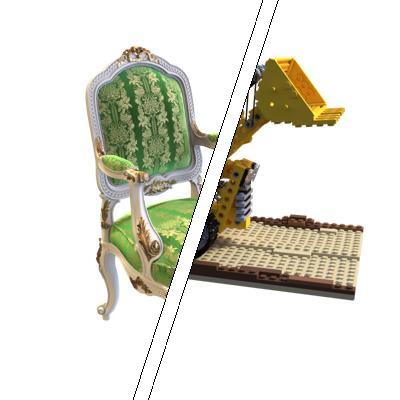
P1 P2 P3
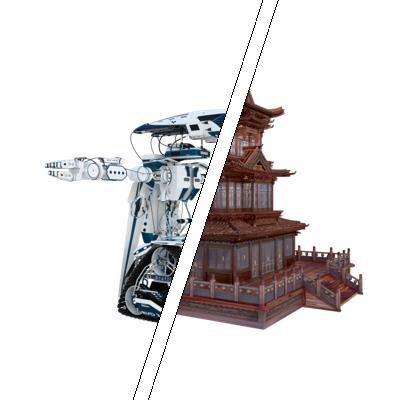
P1 P2 P3
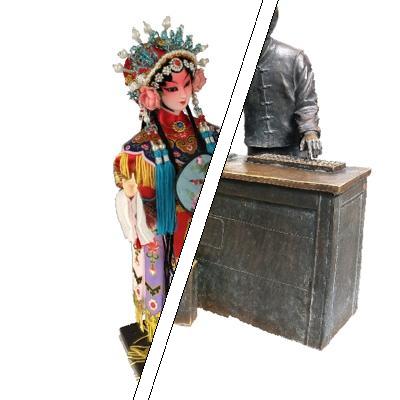
P1 P2 P3
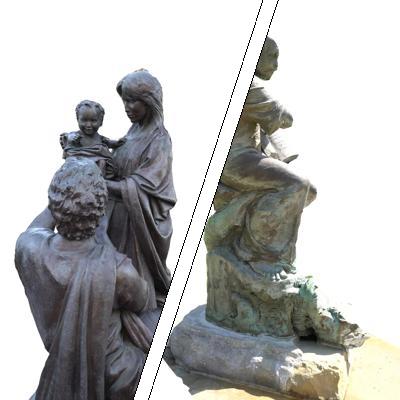
P1 P2 P3
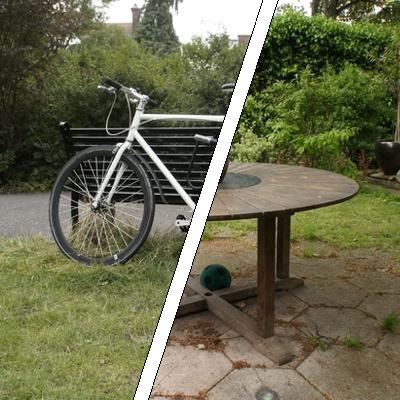
P1 P2 P3